About
As part of the ESA Climate Change Initiative (CCI), the Land Cover project is concerned with the generation of the land cover ECV. Land cover is defined as the physical material at the surface of the earth. Land covers include grass, asphalt, trees, bare ground, water, etc.
The project’s objective is to critically revisit all algorithms required for the generation of a global land product in the light of GCOS requirements, and to design and demonstrate a prototype system delivering in a consistent way over years and from various EO instruments global land cover information matching the needs of key users’ belonging to the climate change community. The focus is placed on the ESA and Member States missions providing near daily global surface reflectance observation at moderate spatial resolution (MERIS FR & RR, SPOT VEGETATION) but the contribution of ESA SAR sensors will also be investigated to tackle specific land cover discrimination issue.
To this aim, the project Phase 1 was subdivided into 3 years.
Year 1. Engagement of the climate modelling community, definition of product specifications and algorithms selection
First, the project defined the detailed specifications of a global land cover product matching the GCOS requirements (both for itself and as a surrogate for other important climate variables), achievable on a regular basis using the current EO systems and building on the FAO/UNEP LCCS for the sake of compatibility with other land cover products. To do so, it engaged with key climate and carbon modelling users to ensure the Land_Cover_cci products meet the requirements for a range of model communities and for application of existing and future modelling approaches. Capitalizing on the GlobCover, GLC2000, GlobAlbedo and GlobCorine experiences, benchmarks and developments of algorithms and methods were carried out to pre-process the various data types according to the state-of-the-art and to discriminate the land cover type in a consistent way over time at global scale.
Year 2: System prototyping and ECV production
After the round robin process and the algorithms selection, the production delivered to the climate community 3 global land cover maps or databases, respectively for the year 2000, for the year 2005 and 2010 both from MERIS FR & RR and SPOT-VGT.
Year 3: Final production and user assessment
The consistency of the land cover information derived from several EO time series currently available at three different epochs were assessed in very detailed manner and the error propagation throughout the prototype system was characterized. The overall and per class accuracy of these new global land cover products were estimated thanks to a widely accepted and independent validation process. The three climate modellers also worked at quantitatively assessing the relevance and usefulness of the delivered information for different climate models. Based on these results, the system specifications for an operational sustainable production of the global land cover information could be defined.
Data
This section provides access to various datasets published by the Land Cover ECV project.
Interactive globe of Land cover class defined in LCCS (1992-01–2015-12) Version: 2.0.7
Products at moderate spatial resolution:
- MRLC maps series from 1992 onwards (v207 and v2.1.1) (300 m)
- Global Plant Functional Type (PFT) map series (300 m)
- Global Water Bodies 4.0 (150 m)
- CCI-LC User Tool
- Land Surface Seasonality Products (500 to 1000 m)
- MERIS surface reflectance time series (300 m)
10-20m demonstration prototypes using Sentinel-2 over Africa and Mesoamerica:
MRLC maps series from 1992 onwards (v207 and v2.1.1)
Consistent global annual land cover maps at 300m spatial resolution from 1992 to 2020
Each pixel value corresponds to the label of a land cover class defined based on the UN Land Cover Classification System (LCCS). LCCS classifiers support the further conversion into Plant Functional Types distribution required by the Earth System Models. The typology counts 22 global land cover categories (see the complete CCI MRLC legend).
The MRLC maps series is delivered along with 4 quality flags documenting the full-time series (they are not year-specific):
- qualityflag1: pixel has been processed or not
- qualityflag2: pixel status as defined by the pre-processing
- qualityflag3: number of valid observations available to derive the classification
- qualityflag4: number of land cover changes detected over the entire period
These maps are derived from a unique baseline MRLC map which is generated thanks to a classification chain applied to the entire MERIS FR and RR archive from 2003 to 2012.
Independently from this baseline, MRLC changes are detected at 1 km based on a time series of annual global classifications generated from AVHRR HRPT (1992 - 1999), SPOT-Vegetation (1999 - 2012) and PROBA-V (2013 - 2015), and Sentinel-3 (recent years). A systematic analysis of the temporal trajectory of each pixel revealed significant land cover changes for a simplified land cover typology matching the IPCC classes: cropland, forest, grassland, wetlands, settlements and other lands. The last category is further split into shrubland, sparse vegetation, bare area and water.
From 2003 onwards, the changes detected at 1km are re-mapped at 300 m. The last step consists of back- and updating the 10-year baseline MRLC map to produce the annual MRLC maps over the entire period.
Some remarks:
- The land cover maps until 2015 (v207) were generated under the ESA CCI umbrella. From 2016 onwards, the maps were operationally generated under the EC Copernicus Climate Change Service.
- The annual MRLC maps v2.0.7 for years 2000, 2005 and 2010 replace completely the "v1.6.1 epoch-based" dataset as the annual MRLC maps v2.0.7 have been improved both in the representation of the areas stable over time and in the characterization of change.
Global Plant Functional Type (PFT) map series
Spatially explicit vegetation fractions for climate models
The PFT global dataset 1992-2020 has 14 layers per year, each describing the percentage cover (0-100%) of a plant functional types at a spatial resolution of 300 m: broadleaved evergreen trees, broadleaved deciduous trees, needleleaved evergreen trees, needleleaved deciduous trees, broadleaved evergreen shrubs, broadleaved deciduous shrubs, needleleaved evergreen, needleleaved deciduous shrubs, shrubs natural grasses, herbaceous cropland (i.e., managed grasses), built, water, bare areas, and snow and ice.
“Plant Functional Types” (PFTs) refer to globally representative and similarly behaving plant types. PFTs can be related to physiognomy and phenology, climate (which defines the geographical ranges in which a plant type can grow and reproduce under natural conditions, and physiological activity (e.g., C3/C4 photosynthetic pathways).
All terrestrial zones of the Earth between the parallels 90°N and 90°S are covered. The PFT dataset has a regular latitude-longitude grid with a grid spacing of 0.002777777777778°, corresponding to ~300 m at the equator and ~200 m in the mid-latitudes. The projection is a Plate-Carrée with a geographic latitude-longitude representation based on the WGS84 ellipsoid.
The plant functional type (PFT) distribution was created by combining auxiliary data products with the CCI MRLC map series. The LC classification provides the broad characteristics of the 300 m pixel, including the expected vegetation form(s) (tree, shrub, grass) and/or abiotic land type(s) (water, bare area, snow and ice, built-up) in the pixel. For some classes, the class legend specifies an expected range for the fractional covers of the contributing PFTs and broadly differentiates between natural and cultivated vegetation. We used a quantitative, globally consistent method that fuses the 300-meter MRLC product with a suite of existing high-resolution datasets to develop spatially explicit annual maps of PFT fractional composition at 300 m. The new PFT product exhibits intraclass spatial variability in PFT fractional cover at the 300-meter pixel level and is complementary to the MRLC maps since the derived PFT fractions maintain consistency with the original LC class legend.
This Data Set was generated to reduce the cross-walking component of uncertainty by adding spatial variability to the PFT composition within a land cover category. This work moved beyond fine-tuning the cross-walking approach for specific LC classes or regions and, instead, separately quantified the PFT fractional composition for each 300 m pixel globally. The result is a dataset representing the cover fractions of 14 PFTs at 300 m, consistent with the CCI MRLC LC maps for the corresponding year.
Citation Harper, K. L., Lamarche, C., Hartley, A., Peylin, P., Ottlé, C., Bastrikov, V., ... & Defourny, P. (2022). A 29-year time series of annual 300-metre resolution plant functional type maps for climate models. Earth System Science Data Discussions, 1-37. https://essd.copernicus.org/preprints/essd-2022-296/
Global Water Bodies 4.0
A global map of open permanent inland water bodies, ocean and land at 150 m spatial resolution.
The CCI WB v4.0 is composed of two layers:
- A static map of open water bodies at 150 m spatial resolution resulting from a compilation and editions of land/water classifications: the Envisat ASAR water bodies indicator, a sub-dataset from the Global Forest Change 2000 - 2012 and the Global Inland Water product. Legend: 1-Land, 2-Water.
This map is delivered at 150 m as a stand-alone product but it is consistent with the class "Water Bodies" of the baseline map used as input to generate the annual LC Maps after resampling to 300 m using an average algorithm. - A static map with the distinction between ocean and inland water is available at 150 m spatial resolution. Legend: 0-Ocean, 1-Land. It is fully consistent with the CCI WB-Map v4.0 at 150 m.
Citation Lamarche, C., Santoro, M., Bontemps, S., d’Andrimont, R., Radoux, J., Giustarini, L., ... & Arino, O. (2017). Compilation and validation of SAR and optical data products for a complete and global map of inland/ocean water tailored to the climate modelling community. Remote Sensing, 9(1), 36. https://www.mdpi.com/2072-4292/9/1/36
CCI-LC User Tool
A user tool dedicated to climate modellers
This tool fits land cover products to climate modellers' needs by sub-setting, resampling, re-projecting and converting land cover classes into Plant Functional Types according to default or user-defined cross-walking tables.
Functionalities:
- Ranking of LC class by fractional area in target cell; first n entries of the sorted list are written to n bands (n is user parameter, called majority classes),
- Fractional area of each LC class,
- Fractional area of each PFT.
Note that the current version of the user tool (v3.3) is not working with the CCI-LC Water Bodies product yet. This will be included in the next user tool version and this is the reason why it can still be fine-tuned.
More info here: http://maps.elie.ucl.ac.be/CCI/viewer.
For any further questions or concerns, email us at contact@esa-landcover-cci.org.
Land Surface Seasonality Products
Three global climatological 7-day time series describing the natural variability of the vegetation, the snow cover and the burned areas
On a per-pixel basis, these LC seasonality products reflect, along the year, the average dynamics and the inter-annual variability of the land surface over the 1998-2012 period. They are expressed as 7-day time profiles of the average and standard deviation for the vegetation greenness (NDVI) or as temporal series of occurrence probabilities for the snow and the burned areas.
Although they are built from existing and independent datasets, they were found to be quite consistent among themselves and with the land cover classes. These products are complementary to the three global CCI-LC maps products characterizing the same period.
Each climatology product is delivered in 52 files (1 file per 7-day time interval) and each file is made of measurements and quality flags.
More info here: http://maps.elie.ucl.ac.be/CCI/viewer.
For any further questions or concerns, contact us at contact@esa-landcover-cci.org.
MERIS surface reflectance time series
The surface reflectance (SR) products consist of MERIS global time series covering the 2003-2012 period. The spectral content encompasses the 13 surface reflectance channels – the atmospheric bands 11 and 15 being removed – and the spatial resolution is of 300 m for the Full Resolution (FR) and 1000 m for the Reduced Resolution (RR). The time series are made of temporal syntheses obtained over a 7-day compositing period. To simplify the handling and analysis of global datasets, the MERIS SR time series are delivered in 5°x5° tiles.
Pre-processing: The pre-processing chain generates global SR time series by a series of pre-processing steps, including radiometric corrections, geometric correction, pixel identification, atmospheric correction with aerosol retrieval, BRDF corrections as well as compositing and mosaicking.
Quality control of input products: The MERIS dataset is very valuable and the use of the full mission dataset in the CCI-LC project in a consistent manner is a major effort. It requires advanced techniques for the development of specific quality checks related to the input data
The quality of each global multispectral SR composite is described, on a per-pixel basis, by a set of flags and values: uncertainties for each spectral band, the current status of the surface, uncertainties for each spectral band, the number of observations with clear sky land coverage, water coverage, clear sky snow and ice coverage, cloudy coverage and cloud shadow coverage for each pixel. The uncertainties of the surface directional reflectance value are calculated from the contributions of each error source, assuming a negligible correlation between the different error sources.
The obtained values are compared with in-situ data from CEOS LandNet sites and with reflectance products available from other sensors and other projects. Besides assessing the quality of individual composites, the quality of the global SR time series is also documented, to quantify their discrimination potential.
More info here: http://maps.elie.ucl.ac.be/CCI/viewer.
CCI-LC Prototypes using Sentinel 2
These were generated within the CCI MRLC initiative using Sentinel-2 data at 20m over the whole of Africa and at 10m over Mesoamerica.
Key Documents
Team
The following partners are part of the Land Cover CCI team:
- Université catholique de Louvain (UCLouvain), Belgium
- Brockmann Consult (BC), Germany
- Met Office - Hadley Centre (MOHC), United Kingdom
- Laboratoire des Sciences du Climat et l'Environnement (LSCE), France
The Land Cover CCI consortium is organised into three main pillars or areas of expertise of equal importance in terms of success for the project:
- EO sciences team pillar, under the leadership of UCLouvain. This pillar includes developing the Land Cover ECV product, including preprocessing by BC, land cover classification, and Plant Functional Types retrieval by UCLouvain. Methodological developments relate to validating land cover change and estimating the end-to-end uncertainty budget led by UCLouvain and BC, respectively.
- Climate Research Group pillar, under the coordination of the LSCE and the MOHC. This pillar includes the evaluation of the impact of using the PFT map series over the conventional maps based on a generic cross-walking table in two land surface models - ORCHIDEE and JULES.
- System engineering pillar, under the responsibility of Brockmann Consult. This pillar includes the translation of the results into analysis-ready datasets.
In support of these pillars, the management activity is operated by UCLouvain.
Since the beginning of the CCI, the Land Cover consortium has also worked closely with other partners: Max Planck Institute for Meteorology (MPI-M), Germany; Gamma Remote Sensing (Gamma), Switzerland, Joint Research Centre (JRC), Italy; University of Pavia (UPavia), Italy; Centre de Recherche Gabriel Lippmann (CRP), Luxemburg; University of Jena (UJENA), Germany, and Wageningen University (WUR), The Netherlands
User Groups
Several actors and types of users can be identified as representatives of the modelling communities concerned with climate and climate change issues. Potential users that define model requirements originate from groups specialized in different fields of science: e.g., working in weather prediction, Global Circulation Modeling (GCM), Regional Climate Modeling (RCM), Global and Regional Earth System modelling, Carbon Cycle Modeling, Dynamic Vegetation and Hydrology modelling and others.
Potential users of the new land cover products are also model development application groups working in numerical weather prediction (NWP), GCMs and RCMs, global and regional Earth System models, carbon cycle models and dynamic vegetation and hydrology models.
From this large array of different users, model usage can be conceptualized at different levels of model engagement in the project:
- Key user group. They are central to all phases of the user interaction within the project and are an integral part, as partners, of the project. They are the Max Planck Institute for Meteorology (MPI-M), the Laboratoire des Sciences du Climat et de l’Environnment (LSCE) and the Meteorological Office Hadley Center (MOHC). Key users are directly involved in the product specifications, product assessment and the final user assessment of the product.
- Associated user group. These users may be participating in meetings and in the user survey and their input would feed into the product specifications. These users are not partners in the project directly but could be engaged, in particular, if involved in the user assessment of the products.
- Broad user community. It will be considered through the information of the project through the World Wide Web and through reviewing scientific literature, participation in meetings to synthesize requirements for the product specifications and receiving feedback from general global land cover data users. This group of users would also include known user requirements coming not directly from climate modellers but the “climate concerned” users such as those making use of land cover information for other societal benefits or national reporting and accounting.
Publications
Click on the following links for publications relating to the Land Cover project.
2020
Zhuo, L., Dai, Q., Zhao, B., & Han, D. (2020). Soil moisture sensor network design for hydrological applications. Hydrology and Earth System Sciences, 24(5), 2577-2591. (DOI: https://doi.org/10.5194/hess-24-2577-2020).
Guzinski, R., Nieto, H., Sandholt, I., & Karamitilios, G. (2020). Modelling High-Resolution Actual Evapotranspiration through Sentinel-2 and Sentinel-3 Data Fusion. Remote Sensing, 12(9), 1433. (DOI: https://doi.org/10.3390/rs12091433).
Karthikeyan, L., Chawla, I., & Mishra, A. K. (2020). A Review of Remote Sensing Applications in Agriculture for Food Security: Crop Growth and Yield, Irrigation, and Crop Losses. Journal of Hydrology, 124905. (DOI: https://doi.org/10.1016/j.jhydrol.2020.124905).
Chirachawala, C., Shrestha, S., Babel, M.S., Virdis, S.G. and Wichakul, S., 2020. Evaluation of global land use/land cover products for hydrologic simulation in the Upper Yom River Basin, Thailand. Science of The Total Environment, 708, p.135148. (DOI: https://doi.org/10.1016/j.scitotenv.2019.135148).
Deng, Y., Wang, S., Bai, X., Luo, G., Wu, L., Cao, Y., Li, H., Li, C., Yang, Y., Hu, Z. and Tian, S., 2020. Variation trend of global soil moisture and its cause analysis. Ecological Indicators, 110, p.105939. (DOI: https://doi.org/10.1016/j.ecolind.2019.105939).
Duveiller, G., Caporaso, L., Abad-Viñas, R., Perugini, L., Grassi, G., Arneth, A. and Cescatti, A., 2020. Local biophysical effects of land use and land cover change: towards an assessment tool for policy makers. Land Use Policy, 91, p.104382. (DOI: https://doi.org/10.1016/j.landusepol.2019.104382).
Huang, H., Wang, J., Liu, C., Liang, L., Li, C. and Gong, P., 2020. The migration of training samples towards dynamic global land cover mapping. ISPRS Journal of Photogrammetry and Remote Sensing, 161, pp.27-36. (DOI: https://doi.org/10.1016/j.isprsjprs.2020.01.010).
Li, C., Li, M., Liu, J., Li, Y. and Dai, Q., 2020. Comparative Analysis of Seasonal Landsat 8 Images for Forest Aboveground Biomass Estimation in a Subtropical Forest. Forests, 11(1), p.45.(DOI: https://doi.org/10.3390/f11010045).
Masson, V., Heldens, W., Bocher, E., Bonhomme, M., Bucher, B., Burmeister, C., de Munck, C., Esch, T., Hidalgo, J., Kanani-Sühring, F. and Kwok, Y.T., 2020. City-descriptive input data for urban climate models: Model requirements, data sources and challenges. Urban Climate, 31, p.100536.(DOI: https://doi.org/10.1016/j.uclim.2019.100536).
Nieves, J.J., Sorichetta, A., Linard, C., Bondarenko, M., Steele, J., Stevens, F., Gaughan, A.E., Carioli, A., Clarke, D., Esch, T. and Tatem, A.J., 2020. Annually modelling built-settlements between remotely-sensed observations using relative changes in subnational populations and lights at night. Computers Environment and Urban Systems, 80, (DOI: https://doi.org/10.1016/j.compenvurbsys.2019.101444).
Qiu, T., Song, C., Zhang, Y., Liu, H. and Vose, J.M., 2020. Urbanization and climate change jointly shift land surface phenology in the northern mid-latitude large cities. Remote Sensing of Environment, 236, p.111477. (DOI: https://doi.org/10.1016/j.rse.2019.111477).
Radoux, J., Waldner, F. and Bogaert, P., 2020. How response designs and class proportions affect the accuracy of validation data. Remote Sensing, 12(2), p.257. (DOI: https://doi.org/10.3390/rs12020257).
Somkuti, P., Bösch, H., Feng, L., Palmer, P.I., Parker, R.J. and Quaife, T., 2020. A new space-borne perspective of crop productivity variations over the US Corn Belt. Agricultural and Forest Meteorology, 281, p.107826. (DOI: https://doi.org/10.1016/j.agrformet.2019.107826)
Soto-Navarro, C., Ravilious, C., Arnell, A., de Lamo, X., Harfoot, M., Hill, S.L.L., Wearn, O.R., Santoro, M., Bouvet, A., Mermoz, S. and Le Toan, T., 2020. Mapping co-benefits for carbon storage and biodiversity to inform conservation policy and action. Philosophical Transactions of the Royal Society B, 375(1794), p.20190128. (DOI: https://doi.org/10.1098/rstb.2019.0128).
Weiss, M., Jacob, F. and Duveiller, G., 2020. Remote sensing for agricultural applications: A meta-review. Remote Sensing of Environment, 236, p.111402.
Zhang, C., Ye, Y., Fang, X., Li, H. and Zheng, X., 2020. Coincidence Analysis of the Cropland Distribution of Multi-Sets of Global Land Cover Products. International Journal of Environmental Research and Public Health, 17(3), p.707. (DOI: https://doi.org/10.3390/ijerph17030707).
2019
Chen, M., Vernon, C.R., Huang, M., Calvin, K.V. and Kraucunas, I.P., 2019. Calibration and analysis of the uncertainty in downscaling global land use and land cover projections from GCAM using Demeter (v1. 0.0). Geoscientific Model Development, 12(5), pp.1753-1764.
De Alban, J.D.T., Prescott, G.W., Woods, K.M., Jamaludin, J., Latt, K.T., Lim, C.L., Maung, A.C. and Webb, E.L., 2019. Integrating Analytical Frameworks to Investigate Land-Cover Regime Shifts in Dynamic Landscapes. Sustainability, 11(4), p.1139.
Estoque, R.C., Ooba, M., Avitabile, V., Hijioka, Y., DasGupta, R., Togawa, T. and Murayama, Y., 2019. The future of Southeast Asia's forests. Nature Communications, 10, pp.1-12.
Fehri, R., Khlifi, S. and Vanclooster, M., 2019. Disaggregating SDG-6 water stress indicator at different spatial and temporal scales in Tunisia. Science of The Total Environment, 694, p.133766. (DOI: https://doi.org/10.1016/j.scitotenv.2019.133766).
Forkel, M., Andela, N., P Harrison, S., Lasslop, G., Van Marle, M., Chuvieco, E., Dorigo, W., Forrest, M., Hantson, S., Heil, A. and Li, F., 2019. Emergent relationships with respect to burned area in global satellite observations and fire-enabled vegetation models. Biogeosciences, 16, pp.57-76.
Georgievski, G. & Hagemann, S., 2019. Characterizing uncertainties in the ESA-CCI land cover map of the epoch 2010 and their impacts on MPI-ESM climate simulations. Theoretical and Applied Climatology, 137 p.1587. (DOI: https://doi.org/10.1007/s00704-018-2675-2).
Guerra, C.A., Rosa, I.M. and Pereira, H.M., 2019. Change versus stability: are protected areas particularly pressured by global land cover change? Landscape Ecology, pp.1-12.
Guidigan, M.L.G., Sanou, C.L., Ragatoa, D.S., Fafa, C.O. and Mishra, V.N., 2019. Assessing Land Use/Land Cover Dynamic and Its Impact in Benin Republic Using Land Change Model and CCI-LC Products. Earth Systems and Environment, 3(1), pp.127-137.
Guo, L., Sun, F., Liu, W., Zhang, Y., Wang, H., Cui, H., Wang, H., Zhang, J., Du, B., 2019. Response of Ecosystem Water Use Efficiency to Drought over China during 1982–2015: Spatiotemporal Variability and Resilience. Forests, 10, p.598.
Hidalgo, J., Lemonsu, A. and Masson, V., 2019. Between progress and obstacles in urban climate interdisciplinary studies and knowledge transfer to society. Annals of the New York Academy of Sciences, 1436(1), pp.5-18.
Ji, Y., Jin, J., Zhu, Q., Zhou, S., Wang, Y., Wang, P., Xiao, Y., Guo, F., Lin, X. and Xu, J., 2019. Unbalanced forest displacement across the coastal urban groups of eastern China in recent decades. Science of The Total Environment, p.135900.
Kganyago, M. and Shikwambana, L., 2019. Assessing Spatio-Temporal Variability of Wildfires and their Impact on Sub-Saharan Ecosystems and Air Quality Using Multisource Remotely Sensed Data and Trend Analysis. Sustainability, 11(23), p.6811. (DOI:https://doi.org/10.3390/su11236811)
Landholm, D.M., Pradhan, P. and Kropp, J.P., 2019. Diverging forest land use dynamics induced by armed conflict across the tropics. Global Environmental Change, 56, pp.86-94.
Li, J., Chen, H., Zhang, C. and Pan, T., 2019. Variations in ecosystem service value in response to land use/land cover changes in Central Asia over 1995-2035 (No. e27645v1). PeerJ Preprints.
Merchant, C.J., Embury, O., Bulgin, C.E., Block, T., Corlett, G.K., Fiedler, E., Good, S.A., Mittaz, J., Rayner, N.A., Berry, D. and Eastwood, S., 2019. Satellite-based time series of sea-surface temperature since 1981 for climate applications. Scientific data, 6(1), pp.1-18.
Mousivand, A. and Arsanjani, J.J., 2019. Insights on the historical and emerging global land cover changes: The case of ESA-CCI-LC datasets. Applied Geography, 106, pp.82-92.
Nowosad, J., Stepinski, T.F. and Netzel, P., 2019. Global assessment and mapping of changes in mesoscale landscapes: 1992–2015. International Journal of Applied Earth Observation and Geoinformation, 78, pp.332-340.
Obu, J., Westermann, S., Bartsch, A., Berdnikov, N., Christiansen, H.H., Dashtseren, A., Delaloye, R., Elberling, B., Etzelmüller, B., Kholodov, A. and Khomutov, A., 2019. Northern Hemisphere permafrost map based on TTOP modelling for 2000–2016 at 1 km2 scale. Earth-Science Reviews, 193, pp.299-316.
Osei, M.A., Amekudzi, L.K., Wemegah, D.D., Preko, K., Gyawu, E.S. and Obiri-Danso, K., 2019. The impact of climate and land-use changes on the hydrological processes of Owabi catchment from SWAT analysis. Journal of Hydrology: Regional Studies, 25, p.100620.
Pohlmann, H., Müller, W.A., Bittner, M., Hettrich, S., Modali, K., Pankatz, K. and Marotzke, J., 2019. Realistic quasi‐biennial oscillation variability in historical and decadal hindcast simulations using CMIP6 forcing. Geophysical Research Letters.
Prósper, M., Sosa Tinoco, I., Otero Casal, C. & Miguez-Macho, G., 2019. Downslope windstorms in the Isthmus of Tehuantepec during Tehuantepecer events: a numerical study with WRF high-resolution simulations. Earth System Dynamics, 10, pp. 485-499.
Romijn, E., Coppus, R., De Sy, V., Herold, M., Roman-Cuesta, R.M. and Verchot, L., 2019. Land Restoration in Latin America and the Caribbean: An Overview of Recent, Ongoing and Planned Restoration Initiatives and Their Potential for Climate Change Mitigation. Forests, 10(6), p.510.
Santini, L., Butchart, S.H., Rondinini, C., Benítez‐López, A., Hilbers, J.P., Schipper, A.M., Cengic, M., Tobias, J.A. and Huijbregts, M.A., 2019. Applying habitat and population‐density models to land‐cover time series to inform IUCN Red List assessments. Conservation Biology.
Schepaschenko, D., See, L., Lesiv, M., Bastin, J.F., Mollicone, D., Tsendbazar, N.E., Bastin, L., McCallum, I., Bayas, J.C.L., Baklanov, A. and Perger, C., 2019. Recent Advances in Forest Observation with Visual Interpretation of Very High-Resolution Imagery. Surveys in Geophysics, 40(4), pp.839–862.
Sims, N.C., England, J.R., Newnham, G.J., Alexander, S., Green, C., Minelli, S. and Held, A., 2019. Developing good practice guidance for estimating land degradation in the context of the United Nations Sustainable Development Goals. Environmental Science & Policy, 92, pp.349-355.
Su, L., Li, J., Shi, X. and Fung, J.C., 2019. Spatiotemporal variation in pre‐summer precipitation over South China from 1979 to 2015 and its relationship with urbanization. Journal of Geophysical Research: Atmospheres.
van Vliet, J., 2019. Direct and indirect loss of natural area from urban expansion. Nature Sustainability. (DOI: https://doi.org/10.1038/s41893-019-0340-0).
Vilar, L., Garrido, J., Echavarría, P., Martínez-Vega, J. and Martín, M.P., 2019. Comparative analysis of CORINE and climate change initiative land cover maps in Europe: Implications for wildfire occurrence estimation at regional and local scales. International Journal of Applied Earth Observation and Geoinformation, 78, pp.102-117.
Waldner, F., Schucknecht, A., Lesiv, M., Gallego, J., See, L., Pérez-Hoyos, A., d'Andrimont, R., De Maet, T., Bayas, J.C.L., Fritz, S. and Leo, O., 2019. Conflation of expert and crowd reference data to validate global binary thematic maps. Remote Sensing of Environment, 221, pp.235-246.
Xu, X., Jain, A.K. and Calvin, K.V., 2019. Quantifying the biophysical and socioeconomic drivers of changes in forest and agricultural land in South and Southeast Asia. Global Change Biology, 25(6), pp.2137-2151.
Yebra, M., Scortechini, G., Badi, A., Beget, M.E., Boer, M.M., Bradstock, R., Chuvieco, E., Danson, F.M., Dennison, P., de Dios, V.R. and Di Bella, C.M., 2019. Globe-LFMC, a global plant water status database for vegetation ecophysiology and wildfire applications. Scientific data, 6(1), pp.1-8.
2018
Duveiller, G., Hooker, J., and Cescatti, A., 2018. The mark of vegetation change on Earth’s surface energy balance. Nature communications, 9(1), p.679.
Duveiller, G., Hooker, J., and Cescatti, A., 2018. A dataset mapping the potential biophysical effects of vegetation cover change. Scientific data, 5, p.180014.
Estoque, R.C., Pontius Jr, R.G., Murayama, Y., Hou, H., Thapa, R.B., Lasco, R.D. and Villar, M.A., 2018. Simultaneous comparison and assessment of eight remotely sensed maps of Philippine forests. International Journal of Applied Earth Observation and Geoinformation, 67, pp.123-134.
Hua, T., Zhao, W., Liu, Y., Wang, S. and Yang, S., 2018. Spatial Consistency Assessments for Global Land-Cover Datasets: A Comparison among GLC2000, CCI LC, MCD12, GLOBCOVER and GLCNMO. Remote Sensing, 10(11), p.1846.
Li, W., MacBean, N., Ciais, P., Defourny, P., Lamarche, C., Bontemps, S., Houghton, R. A., and Peng, S., 2018. Gross and net land cover changes in the main plant functional types derived from the annual ESA CCI land cover maps (1992–2015), Earth System Science Data, 10, 219-234, (DOI: https://doi.org/10.5194/essd-10-219-2018).
Liu, X., Yu, L., Si, Y., Zhang, C., Lu, H., Yu, C. and Gong, P., 2018. Identifying patterns and hotspots of global land cover transitions using the ESA CCI Land Cover dataset. Remote sensing letters, 9(10), pp.972-981.
Liu, X., Yu, L., Li, W., Peng, D., Zhong, L., Li, L., Xin, Q., Lu, H., Yu, C. and Gong, P., 2018. Comparison of country-level cropland areas between ESA-CCI land cover maps and FAOSTAT data. International Journal of Remote Sensing, 39(20), pp.6631-6645.
Schmidt, S., Alewell, C. and Meusburger, K., 2018. Change of permanent grasslands extent (1996-2015) and national grassland dataset of Switzerland. Data in brief, 20, pp.1992-1998.
Toté, C., Swinnen, E., Sterckx, S., Adriaensen, S., Benhadj, I., Iordache, M.D., Bertels, L., Kirches, G., Stelzer, K., Dierckx, W. and Van den Heuvel, L., 2018. Evaluation of PROBA-V Collection 1: Refined Radiometry, Geometry, and Cloud Screening. Remote Sensing, 10(9), p.1375.
Wang, L., Bartlett, P., Chan, E. and Xiao, M., 2018, July. Mapping of Plant Functional Type from Satellite-Derived Land Cover Datasets for Climate Models. In IGARSS 2018-2018 IEEE International Geoscience and Remote Sensing Symposium (pp. 3416-3419). IEEE.
2017
Lauer, A., Eyring, V., Righi, M., Buchwitz, M., Defourny, P., Evaldsson, M., Friedlingstein, P., de Jeu, R., de Leeuw, G., Loew, A. and Merchant, C.J., 2017. Benchmarking CMIP5 models with a subset of ESA CCI Phase 2 data using the ESMValTool. Remote Sensing of Environment, 203, pp.9-39.
Lamarche, C., Santoro, M., Bontemps, S., d’Andrimont, R., Radoux, J., Giustarini, L., Brockmann, C., Wevers, J., Defourny, P. and Arino, O., 2017. Compilation and Validation of SAR and Optical Data Products for a Complete and Global Map of Inland/Ocean Water Tailored to the Climate Modeling Community. Remote Sensing, 9, p.36.
Hartley, A.J., MacBean, N., Georgievski, G. and Bontemps, S., 2017. Uncertainty in plant functional type distributions and its impact on land surface models. Remote Sensing of Environment, 203, pp.71-89.
2016
Eberenz, J., Verbesselt, J., Herold, M., Tsendbazar, N.-E., Sabatino, G., Rivolta, G., 2016. Evaluating the Potential of PROBA-V Satellite Image Time Series for Improving LC Classification in Semi-Arid African Landscapes. Remote Sensing, 8, p.987.
Harper, A. B., Cox, P. M., Friedlingstein, P., Wiltshire, A. J., Jones, C. D., Sitch, S., Mercado, L. M., Groenendijk, M., Robertson, E., Kattge, J., Bönisch, G., Atkin, O. K., Bahn, M., Cornelissen, J., Niinemets, Ü., Onipchenko, V., Peñuelas, J., Poorter, L., Reich, P. B., Soudzilovskaia, N. A., and Bodegom, P. V., 2016. Improved representation of plant functional types and physiology in the Joint UK Land Environment Simulator (JULES v4.2) using plant trait information, Geoscientific Model Development, 9, 2415-2440, (DOI: https://doi.org/10.5194/gmd-9-2415-2016).
Herold, M., See, L., Tsendbazar, N.-E. and Fritz, S. 2016. Towards an Integrated Global Land Cover Monitoring and Mapping System. Remote Sensing, 8, p.1036. (DOI: http://www.mdpi.com/2072-4292/8/12/1036).
2015
Poulter, B., MacBean, N., Hartley, A., Khlystova, I., Arino, O., Betts, R., Bontemps, S., Boettcher, M., Brockmann, C., Defourny, P., Hagemann, S., Herold, M., Kirches, G., Lamarche, C., Lederer, D., Ottlé, C., Peters, M., and Peylin, P., 2015. Plant functional type classification for earth system models: results from the European Space Agency's Land Cover Climate Change Initiative, Geoscientific Model Development, 8, pp.2315-2328, (DOI: https://doi.org/10.5194/gmd-8-2315-2015).
2014
Radoux, J., Lamarche, C., Van Bogaert, E., Bontemps, S., Brockmann, C. and Defourny, P., 2014. Automated training sample extraction for global land cover mapping. Remote Sensing, 6(5), pp.3965-3987.
2013
Hollmann, R., Merchant, C.J., Saunders, R., Downy, C., Buchwitz, M., Cazenave, A., Chuvieco, E., Defourny, P., de Leeuw, G., Forsberg, R. and Holzer-Popp, T., 2013. The ESA climate change initiative: Satellite data records for essential climate variables. Bulletin of the American Meteorological Society, 94(10), pp.1541-1552.
Gamba, P. and Lisini, G., 2013. Fast and efficient urban extent extraction using ASAR Wide Swath Mode data. IEEE Journal of Selected Topics in Applied Earth Observation and Remote Sensing, 6(5), 2184-2195, (DOI: 10.1109/JSTARS.2012.2235410).
Ottle, C., Lescure, J., Maignan, F., Poulter, B., Wang, T. and Delbart, N., 2013. Use of various remote sensing land cover products for PFT mapping over Siberia. Earth System Science Data Discussions, 6, pp.255-296.
Santoro, M. and Wegmüller, U., 2013. Multi-temporal synthetic aperture radar metrics applied to map open water bodies. IEEE Journal of Selected Topics in Applied Earth Observations and Remote Sensing, 7(8), pp.3225-3238.
2012
Bontemps, S., Herold, M., Kooistra, L., van Groenestijn, A., Hartley, A., Arino, O., Moreau, I. and Defourny, P., 2012. Revisiting land cover observation to address the needs of the climate modelling community, Biogeosciences, 9, pp.2145-2157
2011
Poulter, B., Ciais, P., Hodson, E., Lischke, H., Maignan, F., Plummer, S. and Zimmermann, N.E., 2011. Plant functional type mapping for Earth System Models. Geoscientific Model Development, 4, pp.1-18, (DOI: 10.5194/gmd-4-1-2011).
Related links
CCI Land Cover products can be downloaded from http://maps.elie.ucl.ac.be/CCI/viewer/index.php (top right red button).
In collaboration with Planetary Visions and CCI Land Cover team, ESA has produced an animation showing how satellite data and the ESA’s CCI programme are mapping land cover around the world. Watch the animation
Contacts
The following people can be contacted within the Land Cover project.
- Scientific leader: Pierre Defourny (Université catholique de Louvain) – Pierre.Defourny@uclouvain.be
- Scientific and Project Manager: Céline Lamarche (Université catholique de Louvain) – celine.lamarche@uclouvain.be
- ESA technical officer: Olivier Arino – clement.albergel@esa.int
- Technical questions: contact@esa-landcover-CCI.org
News and events
Latest news & events
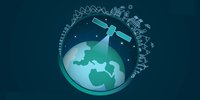
New tender: Tipping elements activity
Latest tender issued as part to the ESA CLIMATE-SPACE programme
Meer leren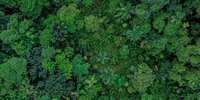
Open Competitive Tender for CLIMATE-SPACE Knowledge Exchange
ESA Tender Action Number: 1-12141. ESA Activity Number: 1000039650.
Meer leren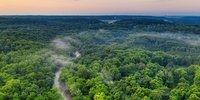
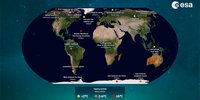
Satellites a ‘clear opportunity’ for forewarning of climate tipping points
New research offers guidance on how remote sensing can help understand and detection tipping systems
Meer leren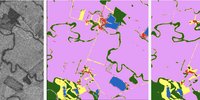
Self-Supervised Learning for SAR; Benchmarking Datasets and Performance on Diverse Downstream Tasks
ESA Climate Office Research Fellow, Dr Anna Jungbluth, presents on 16 Dec at AGU2023
Meer leren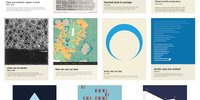
Little Pictures winner announced at COP28
Results of Europe-wide climate data visualisation showcased
Meer leren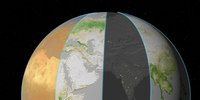
RECCAP2: Global land carbon budget and its attribution to regional drivers
New tender issued by the ESA Climate Office (Activity Number: 1000039196)
Meer leren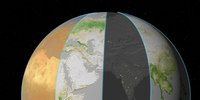
New Tender: CROSS-ECV ACTIVITIES Tender Action Number: 1-12062
New tender issued by the ESA Climate Office (Activity Number: 1000039196)
Meer leren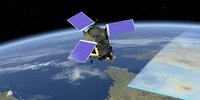
Harnessing Earth Observation for Climate Action
ESA in conversation Prof Jim Skea with IPCC Chair during the COP28 Earth Information day
Meer leren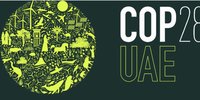
COP28: ESA Climate Office events
The Climate Office is contributing to several events at COP28, UAE, Dubai
Meer leren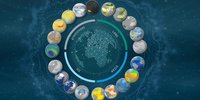
Taking climate action with Earth observation
Satellites' contribution to understanding climate change and supporting climate action are under the COP28 spotlight
Meer leren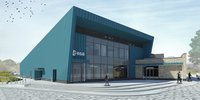